Researchers at Google DeepMind, in collaboration with UC Berkeley, MIT, and the University of Alberta, have developed a new machine learning model to create realistic simulations for training all kinds of AI systems.
“The next milestone for generative models is to simulate realistic experience in response to actions taken by humans, robots, and other interactive agents,” the researchers write. And this is what they hope to achieve with UniSim, a generative AI system that creates a “universal simulator of real-world interaction.”
Although UniSim is in its early stages, it shows the first step toward achieving this milestone. UniSim could prove to be an invaluable asset for fields requiring intricate real-world interactions, such as robotics and autonomous vehicles.
What is UniSim?
UniSim is a generative model that can mimic the interaction between humans and agents with the world. It can simulate the visual outcomes of both high-level instructions, such as “open the drawer,” and low-level controls, like “move by x, y.” This simulated data can then serve as training examples for other models that would need data collection from the real world.
“We propose to combine a wealth of data—ranging from internet text-image pairs, to motion and action rich data from navigation, manipulation, human activities, robotics, and data from simulations and renderings—in a conditional video generation framework,” the researchers write.
According to the researchers, UniSim can successfully merge the vast knowledge contained in its training data and generalize beyond its training examples, “enabling rich interaction through fine-grained motion control of otherwise static scenes and objects.”
UniSim’s ability to simulate realistic experiences has far-reaching implications. It can be used to train embodied planners, low-level control policies, video captioning models, and other machine learning models that demand high-quality and consistent visual data.
Bringing diverse data sources together
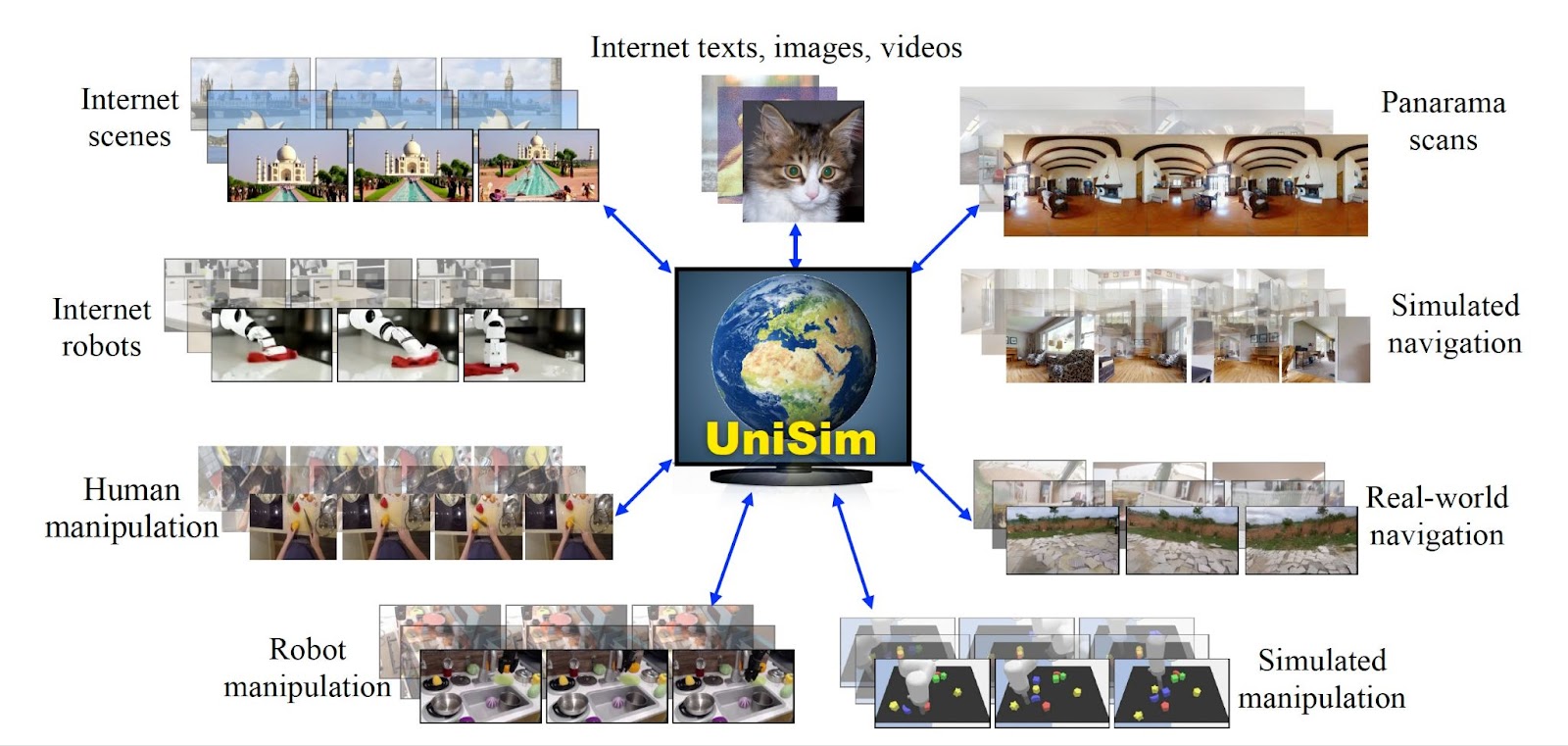
UniSim was trained on dataset gathered from simulation engines, real-world robot data, human activity videos, and image-description pairs. However, the diversity of data formats posed a great challenge to training the model.
“Since different datasets are curated by different industrial or research communities for different tasks, divergence in information is natural and hard to overcome, posing difficulties to building a real-world simulator that seeks to capture realistic experience of the world we live in,” the researchers write.
These datasets have been labeled differently and serve distinct purposes. For instance, paired text-image data offers rich scenes and objects but lacks movement.
Video captioning and question-answering data provide high-level activity descriptions but offer little detail on low-level movement.
Human activity data is rich in human action but lacks mechanical motion, and robotics data, while rich in robot action, is limited in quantity.
To address this challenge, the researchers first converted all the disparate datasets into a unified format. They employed transformer models, the deep learning architecture used in large language models, to create embeddings from text descriptions and non-visual modalities such as motor controls and camera angles. They trained a diffusion model to encode the visual observations that depict the actions. They then conditioned the diffusion model to the embeddings, connecting observations, actions, and outcomes.
Once trained, UniSim can generate a wide range of photorealistic videos, including people performing actions and navigation of environments.
It can also execute long-horizon simulations, such as a robot hand performing a sequence of multiple actions. The generated examples demonstrate that UniSim successfully preserves the structure of the scene and the objects it contains in these long-horizon simulations.
Furthermore, UniSim can generate “stochastic environment transitions,” such as revealing different objects under a cloth or towel. This ability is particularly useful when simulating counterfactuals and different scenarios in computer vision applications.
Bridging the sim-to-real gap
UniSim’s ability to generate realistic videos from text descriptions is remarkable, but its true value lies in integration with reinforcement learning environments. Here, UniSim can simulate various outcomes in applications such as robotics, enabling offline training of models and agents without the need for real-world training.
The researchers highlight the benefits of this approach: “Using UniSim as an environment to train policies has a few advantages including unlimited environment access (through parallelizable video servers), real-world like observations (through photorealistic diffusion outputs), and flexible temporal control frequencies (through temporally extended actions across low-level robot controls and high-level text actions).”
Simulation environments are a staple in reinforcement learning. However, UniSim’s high visual quality can help diminish the disparity between learning in simulation and in the real world, a challenge often referred to as the “sim-to-real gap.”
According to the researchers, models trained with UniSim “can generalize to real robot settings in a zero-shot manner, achieving one step towards bridging the sim-to-real gap in embodied learning.”
Applications of UniSim
A real-world simulator like UniSim has many potential applications, spanning from controllable content creation in games and movies to training embodied agents purely in simulation for direct deployment in the real world. UniSim can also complement the advances in vision language models (VLM) such as DeepMind’s recent RT-X models.
VLM agents require substantial real-world data, particularly when executing complex, multi-step tasks. The researchers demonstrate that UniSim can generate large volumes of training data for VLM policies.
“We use UniSim to train both high-level vision-language planners and low-level reinforcement learning policies, each of which exhibit zero-shot real-world transfer after training purely in a learned real-world simulator,” the researchers state. This approach extends to other types of models, such as video captioning models, which can benefit from training with simulated experience in UniSim.
UniSim can also simulate rare events, a feature that is particularly useful in robotics and self-driving car applications, where data collection can be costly and risky.
The researchers acknowledge that “UniSim requires large compute resources to train similar to other modern foundation models.” According to the paper, the model required 512 Google TPU-v3 chips during training. “Despite this disadvantage,” the researchers note, “we hope UniSim will instigate broad interest in learning and applying real-world simulators to improve machine intelligence.”
TechForgePulse's mission is to be a digital town square for technical decision-makers to gain knowledge about transformative enterprise technology and transact. Discover our Briefings.